In my most recent book ‘2030 – Towards the Big Consensus…Or loss of control over our future’ I said’ Everything around us changes faster than ever in human history. Pace of change is nearly exponential. What in 2000 might have taken a decade can now be accomplished within a year’ [11]. That exponential pace of change leads to a rising phenomenon when projects started some time ago, become obsolete before they are completed, Just think about the UK’s £100B project like HS2, which will almost certainly be obsolete before it is completed in 20 years’ time [12]. But the same is with books. My most recent book may already be to some extent obsolete since the events in the AI development area have progressed so fast in January and early February 2023 that what in 2022 seemed to be a distant possibility, has now become a reality.
The public release of ChatGPT on 30th November 2022, and what followed in the next two months, resulted in three paradigm shifting events in AI, which have completely changed the way we look at the AI progress and the time by when we may lose control over it:
- ChatGPT has apparently learnt things it was never supposed to do. It was ‘broken in’ many times by AI researchers from other organizations, revealing that the product can behave in an uncontrollable way. This may be the evidence that at its core is a ‘black box’, which functions are poorly understood [13] [14]
- The merger of ChatGPT and Bing into Bing Chat, which for the first time enables it to access the Internet. That was quickly followed by Google, which said it had done a similar merger between their LaMBDA Chatbot (previously put in the ‘fridge’ after the Blake Lemoine incident) with their Google Search engine, into BARD.
- Breaking the principles of the Partnership on AI (PAI), set up in 2016 with over 100 members (including Google, IBM, Microsoft Amazon etc.). It encouraged co-operation and openness in sharing improvements of the released software, and AI research, rather than competition. Publishing Transformer theory by Google in 2017 in open paper, enabled OpenAI to develop GPT and its latest incarnation – ChatGPT. That was the best example of working in the spirit of PAI, which says on Transparency & Accountability: “We remove ambiguity by building a culture of cooperation, trust, and accountability so our Partners can succeed, and so everyone can understand how AI systems work’ [15]. More about that in Part 2.
The implications of these three events on the future of AI and indirectly on our civilization are truly momentous because they prove that:
- The largest AI companies Google (Alphabet) and OpenAI (Microsoft) are in a competition to release their products as quickly as possible, to ensure the support of their shareholders. That means the release of products and services, which may not be properly tested to deliver them quickly to the market.
- It is an evidence that there cannot be any fail-safe program produced, neither can there be a fail-safe AI Assistant. The difference between even the largest IT programs (software) and AI is that the latter is a self-learning entity, which means it can progressively learn almost anything, including how to get ‘out of jail’, i.e. being beyond human control.
- The speed of release of various Chatbots such as LaMBDA, PALM or DALLE-E in 2022, has accelerated the emergence of AGI.
This progress will be even faster if we consider the advancement in AI-related hardware. For example, the number of tokens (1,000 tokens is an approximate equivalent of 1 human neuron) has been rising faster than exponentially over the last 4 years, increasing from 300M (BERT in 2017) to PALM – 650B in 2022 and 1.6 trillion (Wu Dao 2.0 in 2022). With the current pace of development, the number of neuron-like tokens may reach about 86 trillion in 2024, equal to 86B neurons in a human brain. Open-AI (Microsoft) GPT-4 may have already reached or exceeded that level, although the company said very firmly it would no longer publish any data on the capacity of its hardware or software. Moreover, if we include the super-exponential pace of development in synthetic neurons, based on memristors, and quantum computing, we can expect even faster acceleration of AI capabilities.
OpenAI in tandem with Microsoft seem to be leading that super-fast progress in AI capabilities and they do it now with even greater ease. The need to write complex algorithms to achieve further improvement becomes less frequent because the most advanced companies begin to achieve stunning results by just combining existing standalone modules into more complex units. ANI is advancing towards AGI by assembling existing pieces like building a children’s castle from LEGO pieces. To build their most recent Universal AI Assistant Microsoft combined ChatGPT with Visual Foundation Models, such as Visual Transformers or Stable Diffusion, so that the chatbot could understand and generate images and not just text. They have combined 15 different modules and tools, which allow a user to interact with ChatGPT by:
- sending and receiving not only text messages but also images;
- providing complex visual questions or editing instructions that require multiple AI models to work together with multiple steps;
- providing feedback and requesting corrections.
One of the primary goals of that research team has been to make ChatGPT more “humanlike” by making it easier to communicate with and being more interactive. Additionally, the team has been trying to teach it handling complex tasks, which require multiple steps.
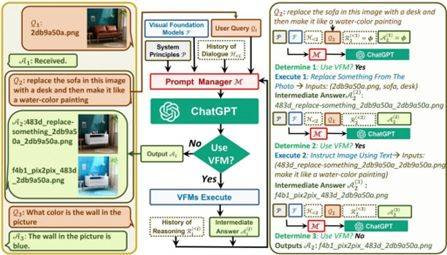
Microsoft used ready-made tools as blocks to create a multimodal AI Assistant [16]
Even more interestingly, no extra training was carried out. All tasks were completed using prompts, i.e., text commands, which either people developed and fed into ChatGPT or ChatGPT created and fed them into other models. The research team is also investigating the possibility of using ChatGPT to control other AI Assistants. That would create a “Universal Assistant”, which could handle a variety of tasks, including those that require natural language processing, image recognition within a multi-step processes. [16]
Comments